Learn how predictive analytics is helping healthcare providers prevent disease outbreaks.
In the age of digital transformation, predictive analytics is emerging as a game-changing tool in healthcare. By analyzing vast amounts of data, predictive analytics enables healthcare providers and public health organizations to anticipate and prevent disease outbreaks before they occur. This proactive approach saves lives, reduces healthcare costs, and enhances global health security. From identifying patterns in disease spread to optimizing resource allocation, predictive analytics is revolutionizing disease prevention.
This article delves into how predictive analytics is preventing disease outbreaks, the technologies driving this shift, and the challenges and opportunities it presents.
What is Predictive Analytics in Healthcare?
Predictive analytics involves the use of statistical techniques, machine learning, and data mining to analyze historical and real-time data, identify trends, and make predictions about future outcomes. In healthcare, predictive analytics is applied to:
- Monitor disease patterns.
- Predict patient outcomes.
- Optimize treatment plans.
- Prevent the spread of infectious diseases.
By leveraging data from various sources—including electronic health records (EHRs), environmental data, and social media—predictive analytics provides actionable insights to mitigate health risks.
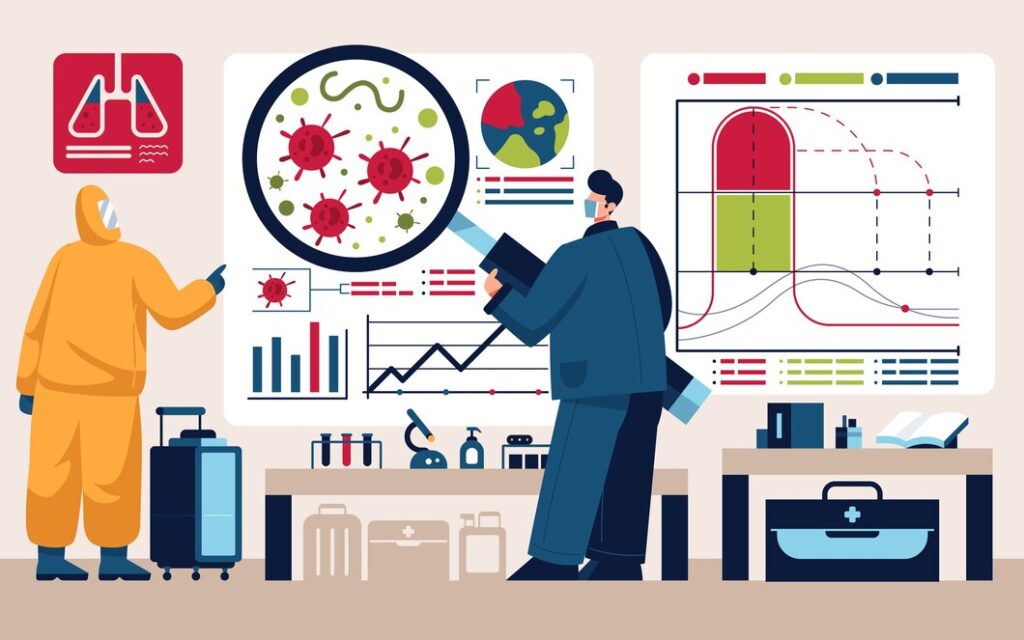
The Role of Predictive Analytics in Preventing Disease Outbreaks
1. Early Detection of Outbreaks
Predictive analytics enables the early detection of disease outbreaks by analyzing data from multiple sources, such as:
- Health Reports: Hospital admissions, laboratory test results, and disease registries.
- Environmental Data: Weather patterns, air quality, and water contamination.
- Social Media and News: Public sentiment and emerging health concerns.
By identifying anomalies or unusual patterns, predictive models can alert healthcare authorities to potential outbreaks, enabling timely interventions.
2. Real-Time Monitoring
Advanced analytics tools continuously monitor data streams to provide real-time insights into disease dynamics. For instance, wearable devices and IoT-enabled sensors collect data on vital signs, which can be analyzed to detect early signs of infection. This real-time monitoring enhances situational awareness and facilitates rapid response.
3. Disease Spread Forecasting
Predictive models simulate the spread of infectious diseases by factoring in variables such as population density, travel patterns, and vaccination rates. Tools like SEIR (Susceptible-Exposed-Infectious-Recovered) models help predict the trajectory of outbreaks, guiding containment strategies.
4. Optimizing Resource Allocation
During an outbreak, predictive analytics helps optimize the allocation of resources such as vaccines, medications, and medical personnel. By forecasting demand, healthcare systems can ensure that critical supplies are available where they are needed most.
5. Enhancing Vaccination Campaigns
Predictive analytics identifies high-risk populations and regions with low vaccination coverage, enabling targeted vaccination campaigns. This approach minimizes the spread of diseases and maximizes the impact of immunization programs.
Applications of Predictive Analytics in Disease Prevention
1. Combatting Seasonal Flu
Predictive analytics models track flu activity by analyzing historical data, weather patterns, and vaccination rates. These insights enable healthcare providers to prepare for flu seasons by stocking vaccines and educating the public.
2. Managing COVID-19
During the COVID-19 pandemic, predictive analytics played a pivotal role in tracking infection rates, predicting hotspots, and evaluating the impact of interventions like social distancing and mask mandates. Real-time dashboards informed policymakers and guided public health strategies.
3. Controlling Vector-Borne Diseases
Diseases like malaria and dengue are influenced by environmental factors. Predictive models analyze weather patterns, mosquito population data, and land use to forecast outbreaks, enabling targeted control measures such as spraying and bed net distribution.
4. Addressing Antibiotic Resistance
Predictive analytics identifies patterns of antibiotic resistance, helping healthcare providers choose effective treatments. By monitoring resistance trends, healthcare systems can implement policies to reduce overuse and misuse of antibiotics.
5. Preventing Hospital-Acquired Infections (HAIs)
Predictive tools monitor infection rates within healthcare facilities, identifying risk factors and recommending preventive measures. This reduces the incidence of HAIs and improves patient outcomes.
Technologies Driving Predictive Analytics
1. Machine Learning and AI
Machine learning algorithms process large datasets to identify patterns and make accurate predictions. These technologies are particularly effective in analyzing complex relationships between variables, such as disease transmission dynamics.
2. Big Data Analytics
The integration of big data enables predictive models to analyze diverse datasets, including EHRs, genomic data, and social determinants of health. This holistic approach enhances predictive accuracy.
3. Cloud Computing
Cloud-based platforms provide the scalability and computational power needed to analyze massive datasets in real time. They also facilitate data sharing among stakeholders, improving collaboration.
4. Geographic Information Systems (GIS)
GIS tools visualize disease patterns by mapping outbreak data. These visualizations help public health officials identify hotspots and implement location-specific interventions.
Benefits of Predictive Analytics in Disease Prevention
1. Proactive Healthcare
Predictive analytics shifts healthcare from a reactive to a proactive model, enabling early interventions that prevent disease spread and reduce mortality rates.
2. Cost Savings
By preventing outbreaks and optimizing resource allocation, predictive analytics reduces healthcare costs associated with hospitalizations and emergency responses.
3. Improved Public Health
Predictive tools enhance public health initiatives by providing data-driven insights for policy development, vaccination campaigns, and health education.
4. Enhanced Global Health Security
In a connected world, disease outbreaks can quickly become global threats. Predictive analytics strengthens global health security by enabling coordinated responses to emerging threats.
Challenges in Implementing Predictive Analytics
1. Data Quality and Accessibility
Accurate predictions require high-quality data from diverse sources. Data gaps, inconsistencies, and limited accessibility can hinder predictive analytics efforts.
2. Privacy and Security Concerns
The use of sensitive health data raises privacy and security concerns. Compliance with regulations like HIPAA and GDPR is essential to maintain public trust.
3. Interoperability Issues
Healthcare systems often lack interoperability, making it difficult to integrate data from multiple sources. Standardizing data formats and protocols is critical for effective predictive analytics.
4. Ethical Considerations
Predictive analytics must be used ethically to avoid unintended consequences, such as discrimination or stigmatization of high-risk populations. Transparent algorithms and accountability measures are necessary.
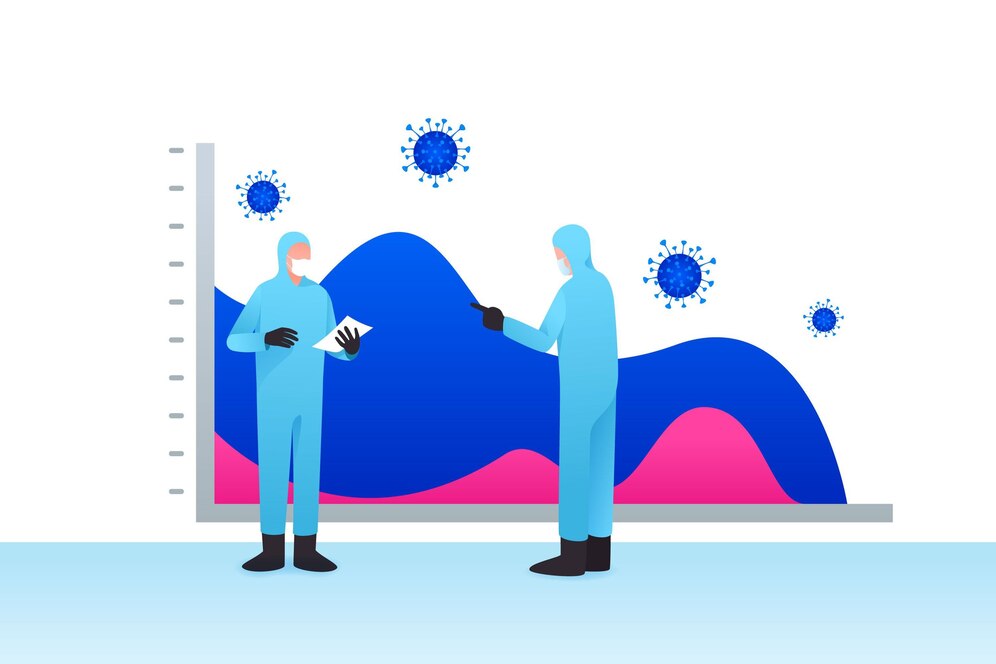
The Future of Predictive Analytics in Disease Prevention
The future of predictive analytics in healthcare is promising, with advancements in technology and data science paving the way for more sophisticated applications. Key trends include:
- Integration of Genomic Data: Incorporating genomic data into predictive models will enable personalized disease prevention strategies.
- AI-Driven Insights: AI algorithms will continue to evolve, providing even more accurate and actionable predictions.
- Global Data Sharing: Collaborative platforms will facilitate global data sharing, enhancing pandemic preparedness and response.
- Real-Time Decision Support: Predictive tools will integrate with clinical decision support systems, providing real-time recommendations to healthcare providers.
As these advancements unfold, predictive analytics will become an indispensable tool in safeguarding public health and preventing disease outbreaks.
Predictive analytics is transforming the fight against disease outbreaks, equipping healthcare providers with the tools to stay ahead of emerging threats. By embracing this technology, the healthcare industry can create a safer and healthier future for all.
FAQs
What is predictive analytics in healthcare?
Predictive analytics uses data analysis, machine learning, and statistical models to predict future health outcomes and trends.
How does predictive analytics prevent disease outbreaks?
It identifies patterns, monitors real-time data, and forecasts disease spread, enabling early interventions and resource optimization.
What types of data are used in predictive analytics?
Data sources include electronic health records, environmental data, social media, and wearable device data.
What role did predictive analytics play during COVID-19?
It tracked infection rates, predicted hotspots, and evaluated the impact of interventions like lockdowns and vaccinations.
What are the benefits of predictive analytics in disease prevention?
Benefits include early detection, cost savings, proactive healthcare, and improved public health initiatives.
What technologies drive predictive analytics?
Key technologies include machine learning, big data analytics, cloud computing, and geographic information systems (GIS).
What challenges does predictive analytics face?
Challenges include data quality issues, privacy concerns, interoperability problems, and ethical considerations.
Can predictive analytics be used for chronic disease management?
Yes, it helps monitor risk factors and predict complications, enabling timely interventions for chronic conditions.
How does predictive analytics optimize vaccination campaigns?
It identifies high-risk populations and regions, ensuring targeted and efficient vaccination efforts.
What is the future of predictive analytics in healthcare?
The future includes AI-driven insights, global data sharing, integration of genomic data, and real-time decision support systems.